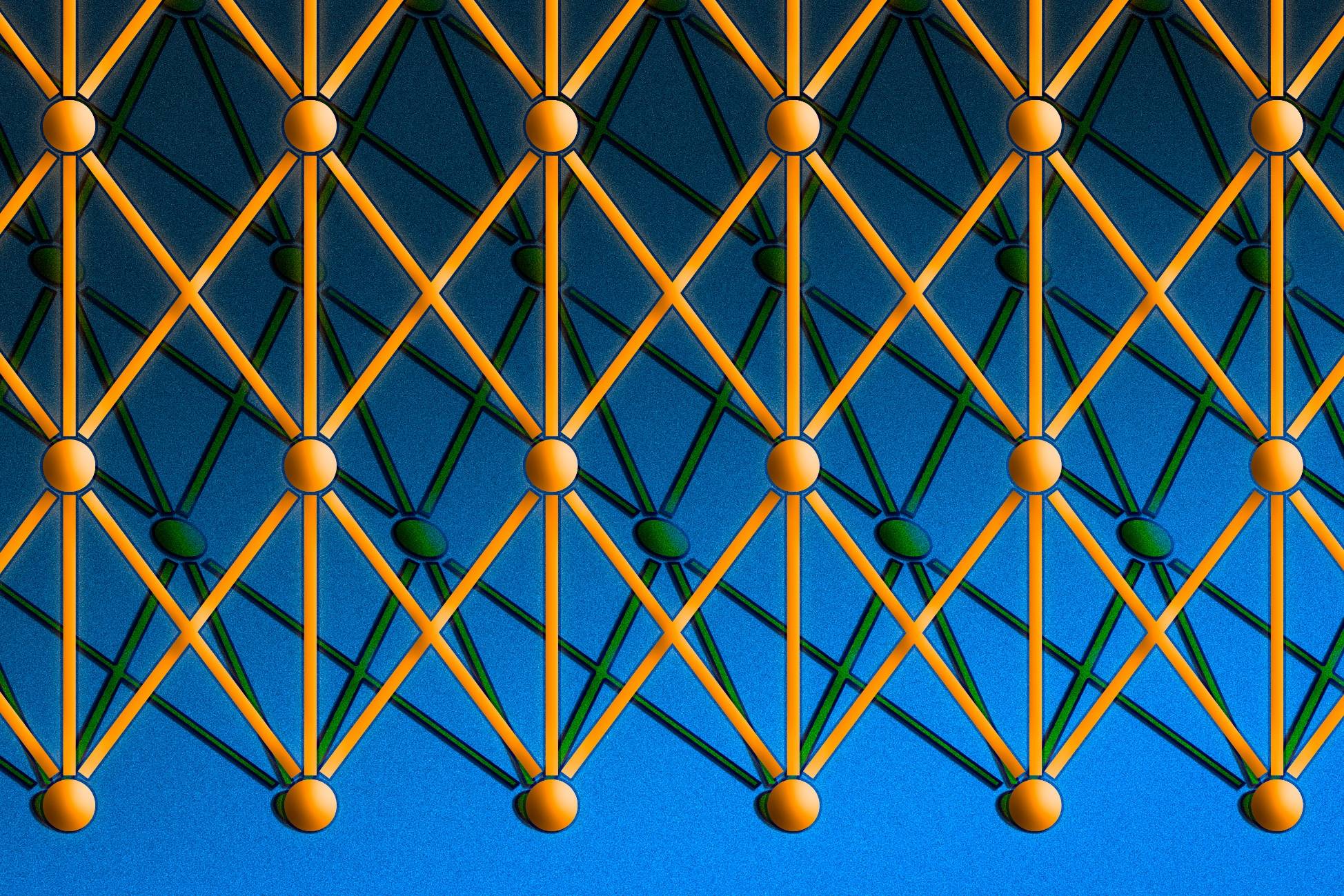
Recent research has highlighted the challenges and opportunities that machine learning fairness presents. It cites a paper titled Co-Designing Checklists to AI Fairness by Michael A. Madaio (Luke Stark), Jennifer Wortman Vaughan and Hanna Wallach. Fairness is crucial in the development AI.
Interview about machine learning fairness
Machine learning is a big topic, but fairness is not given enough attention in concrete projects. Fairness issues are often not considered by most teams, and practitioners know that they do not address them adequately. Generally, fairness work is pushed by activists within the team or by interested individuals who have a personal stake in the issue. But they rarely have the support of the organization they work for.
Fairness efforts are also complicated by issues of data privacy and data reuse. The data collected by machine learning systems is often fixed, limiting the practitioner's ability to influence the outcome. Fairness concerns can be project-specific and it can be difficult for teams to share insights.
Evaluation of equalized odds
It is important to assess the fairness and accuracy of a prediction by using equalized odds. It requires both test and production data. When a predictive model produces equal amounts of correct and wrong predictions, equalized odds can be considered fair. Equalized odds are also known as predictive parity, predictive equality, and predictive inequality. These terms refer generally to fairness in justice and equality, as well disparate treatment.
The following example illustrates how an algorithm that equalizes odds can be used to test this. A university admits a Lilliputian as well as a Brobdingnagian. Brobdingnagians don't have as rigorous secondary schools, so the Lilliputians are more likely to be admitted. Despite having lower odds, Lilliputians have a higher acceptance rate on average than Brobdingnagians.
Machine learning: Barriers to fairness
Fairness work is an important area to consider when designing new systems. Practitioners report that fairness work is rarely a top priority within organizations and that it is almost always reactive. Fairness work typically only takes place when external pressures arise, and resources are limited. Fairness work can often be overlooked because there are no formal requirements.
We can use explainability methods to make sure the models don't have biases. They can be used to identify key model attributes and features that are associated in protected attributes. We can also use model inference methods to evaluate the fairness and validity of a model.
Methods for evaluating machine learning's fairness
The development of fairer machine-learning algorithms can be made easier by developing fair methods to assess fairness. Consider the importance of each feature in the model. When the effect of a feature is larger than expected, the model fails to be fair. Adebayo, et al. showed that gender had low significance in a bank’s credit-limit model. They also found it not to be overly dependent on it.
Predictive models are used for making decisions on a wide variety of topics including employment, access and creditworthiness. This article will discuss five tools that can be used to audit the fairness and validity of these models. FairML is a Python toolbox that quantifies model inputs. It's the first tool. This tool employs four input ranking algorithms to ensure fair predictions.
FAQ
Who invented AI and why?
Alan Turing
Turing was conceived in 1912. His mother was a nurse and his father was a minister. He was an excellent student at maths, but he fell apart after being rejected from Cambridge University. He began playing chess, and won many tournaments. He was a British code-breaking specialist, Bletchley Park. There he cracked German codes.
He died in 1954.
John McCarthy
McCarthy was born on January 28, 1928. He studied maths at Princeton University before joining MIT. The LISP programming language was developed there. He was credited with creating the foundations for modern AI in 1957.
He died on November 11, 2011.
AI: Why do we use it?
Artificial intelligence refers to computer science which deals with the simulation intelligent behavior for practical purposes such as robotics, natural-language processing, game play, and so forth.
AI can also be called machine learning. This refers to the study of machines learning without having to program them.
There are two main reasons why AI is used:
-
To make your life easier.
-
To be better at what we do than we can do it ourselves.
Self-driving vehicles are a great example. AI is able to take care of driving the car for us.
What are some examples AI-related applications?
AI is used in many areas, including finance, healthcare, manufacturing, transportation, energy, education, government, law enforcement, and defense. These are just a few of the many examples.
-
Finance - AI is already helping banks to detect fraud. AI can scan millions of transactions every day and flag suspicious activity.
-
Healthcare – AI helps diagnose and spot cancerous cell, and recommends treatments.
-
Manufacturing - AI can be used in factories to increase efficiency and lower costs.
-
Transportation – Self-driving cars were successfully tested in California. They are being tested across the globe.
-
Utilities are using AI to monitor power consumption patterns.
-
Education - AI is being used in education. Students can interact with robots by using their smartphones.
-
Government - AI can be used within government to track terrorists, criminals, or missing people.
-
Law Enforcement – AI is being utilized as part of police investigation. Investigators have the ability to search thousands of hours of CCTV footage in databases.
-
Defense - AI is being used both offensively and defensively. Offensively, AI systems can be used to hack into enemy computers. Defensively, AI can be used to protect military bases against cyber attacks.
Are there potential dangers associated with AI technology?
Yes. They always will. AI poses a significant threat for society as a whole, according to experts. Others argue that AI can be beneficial, but it is also necessary to improve quality of life.
AI's misuse potential is the greatest concern. It could have dangerous consequences if AI becomes too powerful. This includes autonomous weapons, robot overlords, and other AI-powered devices.
AI could take over jobs. Many people are concerned that robots will replace human workers. Others believe that artificial intelligence may allow workers to concentrate on other aspects of the job.
Some economists even predict that automation will lead to higher productivity and lower unemployment.
Which countries lead the AI market and why?
China has the largest global Artificial Intelligence Market with more that $2 billion in revenue. China's AI industry includes Baidu and Tencent Holdings Ltd. Tencent Holdings Ltd., Baidu Group Holding Ltd., Baidu Technology Inc., Huawei Technologies Co. Ltd. & Huawei Technologies Inc.
The Chinese government has invested heavily in AI development. Many research centers have been set up by the Chinese government to improve AI capabilities. These include the National Laboratory of Pattern Recognition and State Key Lab of Virtual Reality Technology and Systems.
Some of the largest companies in China include Baidu, Tencent and Tencent. These companies are all actively developing their own AI solutions.
India is another country which is making great progress in the area of AI development and related technologies. India's government is currently focusing their efforts on creating an AI ecosystem.
What is the role of AI?
An artificial neural networks is made up many simple processors called neuron. Each neuron processes inputs from others neurons using mathematical operations.
The layers of neurons are called layers. Each layer performs an entirely different function. The raw data is received by the first layer. This includes sounds, images, and other information. Then it passes these on to the next layer, which processes them further. Finally, the output is produced by the final layer.
Each neuron has a weighting value associated with it. This value is multiplied each time new input arrives to add it to the weighted total of all previous values. If the result is greater than zero, then the neuron fires. It sends a signal up the line, telling the next Neuron what to do.
This is repeated until the network ends. The final results will be obtained.
Statistics
- According to the company's website, more than 800 financial firms use AlphaSense, including some Fortune 500 corporations. (builtin.com)
- Additionally, keeping in mind the current crisis, the AI is designed in a manner where it reduces the carbon footprint by 20-40%. (analyticsinsight.net)
- The company's AI team trained an image recognition model to 85 percent accuracy using billions of public Instagram photos tagged with hashtags. (builtin.com)
- In 2019, AI adoption among large companies increased by 47% compared to 2018, according to the latest Artificial IntelligenceIndex report. (marsner.com)
- A 2021 Pew Research survey revealed that 37 percent of respondents who are more concerned than excited about AI had concerns including job loss, privacy, and AI's potential to “surpass human skills.” (builtin.com)
External Links
How To
How to create an AI program that is simple
You will need to be able to program to build an AI program. Although there are many programming languages available, we prefer Python. There are many online resources, including YouTube videos and courses, that can be used to help you understand Python.
Here's a quick tutorial on how to set up a basic project called 'Hello World'.
You'll first need to open a brand new file. For Windows, press Ctrl+N; for Macs, Command+N.
Then type hello world into the box. To save the file, press Enter.
For the program to run, press F5
The program should show Hello World!
This is just the start. These tutorials can help you make more advanced programs.